Post Graduate Certification Program In Data Science
Data Sciences, the Linchpin in emerging Internet Era Accelerate your career by pursuing cutting edge Data Sciences Training at Digital Nest. With the improvements in the field of Automation and Technology, a high influx of techie aspirants are moving towards Data Sciences. Considering the huge demand for the course not only in Hyderabad but also in every corner, Digital Nest initiated an extensive program in Data Sciences.We at Digital Nest, Hyderabad ensure to cater your needs and bridge the gap between academia and industry globally by providing quality education.
4.5 Rating | 1000+ Enrolments | Classroom , Online & E- Learning
Post Graduate Certification Program In Data Science
Data Sciences, the Linchpin in emerging Internet Era Accelerate your career by pursuing cutting edge Data Sciences Training at Digital Nest. With the improvements in the field of Automation and Technology, a high influx of techie aspirants are moving towards Data Sciences. Considering the huge demand for the course not only in Hyderabad but also in every corner, Digital Nest initiated an extensive program in Data Sciences.We at Digital Nest, Hyderabad ensure to cater your needs and bridge the gap between academia and industry globally by providing quality education.
4.5 Rating | 1000+ Enrolments
Classroom , Online & E- Learning
Enquire : 808-899-8664
11 Months
Duration
1000+
Course Enrollments
100%
Placement Assistance
320+
Hours Of Content
0% EMI
3 Partner Banks
Key Highlights Of Course
320+ Learning Hours


5 Projects
15+ Assignments
100% Placements Assistance




Learn From Experts The Most Advanced Post Graduate Program in Data Science
Overview | Curriculum | Faculty | Tools | Certification | Admission Process | Reviews | FAQs
Course Curriculum
Curriculum prepared alumnus of IIT Chennai and reviewed by top notch Data Science experts
1. Introduction to Data Science
- What is Data Science?
- Data Science Life Cycle
- What is Machine Learning?
- What is Business Analytics?
- What is Artificial Intelligence?
- Data Science vs Machine Learning vs AI
- Types of Data
- What is Big Data?
- Software vs Data vs Cloud
- Real time applications on Machine Learning
2. Statistics
- Data Types
- Statistical parameters, variance, standard deviation, range
- Categorical and Quantitative Data
- Descriptive Statistics
- Statistical Inference
- Sampling and Sampling Distributions
- Correlation, Covariance and Causation
- Central Limit Theorem
- Confidence Interval
- Hypothesis Testing and Error Types
- t-test and types of t-test
- Analysis of Variance (ANOVA)
- Introduction to Probability
- Probability Distributions
- Bernoulli, Uniform, Binomial, Normal Distribution
- Poisson and Exponential Distribution
- Skew Normal Distribution
- Z-Score
3. Exploratory Data Analysis(EDA) and Data Visualization
- What is EDA and its Importance
- Statistical approach (Data Collection, Descriptive statistics, Data Mining)
- Importing the Data
- Data Frames
- Variables, Transformation
- Standardization and Normalization
- Validation and Interpretation
- Distributions
- Histograms, Outliers
- Summarizing Distributions
- Graphs
- Bar Charts
- Box-whisker plot
- Scatter plot
- Pie Charts
- Bubble Charts
4. Introduction to R Programming
- Why R and importance of R in Analytics
- Installation of R and R-studio
- Working Directories
- Data Types
- Operators
- Loops-For and While
- If-else statements, Nested statements
- Objects, and Vectors
- Strings
- Arrays
- Lists
- Factors
- Data Frames
- Pipe Operator
- Functions (Predefined and User defined) apply, l-apply, s-apply, m-apply, t-apply, v-apply Subset/filter, which, sample, match, sort, mutate, grep, summary,gsub, select, groupby, gather, separate, Posixct
- Joins (Inner, Outer, Left, Right, Semi, Anti) in Data Frames.
- Univariate Analysis
- Dplyr, Lubridate, Tibble
5. Data manipulation using SQL
- Introduction to SQL and Data bases
- SQL developer installation
- Data types
- Data types and Operators
- Create and Drop data base
- DDL,DML, DCL ,TCL, Sorting commands and other keywords
- Advanced SQL-Wild cards, Constraints, Joins, Unions, NULL, Alias, Truncate, Views, Sub queries
- Exam
6. Introduction to Python Programming
- What is Python?
- Importance of Python in Data Science
- Python Installation Guidelines (Anaconda Navigator)
Python Fundamentals
- Keywords
- Built-in functions
- String Formatting
- Indexing
- Slicing
- Sequences
- Error handling in Python (try, catch, finally)
- Ignoring Warnings
- User-defined functions
- Nested functions
- Lambda, zip and map
Local and global variables
- If-else statements, Nested statements
- Loops, Nested Loops, For, While loop
- Performance measurement of loops
- Loop control statements
- Continue, Break, Pass
- Class, Constructor and methods
Python Data Structures
- Lists, Lists Comprehensions
- Sets
- Tuple
- Dictionary
- Importance of each type
Data Handling with Python
- Introduction to NumPy, Pandas
- Arrays and Matrix
- Importing and exporting datasets in Python
- Creating Data Frames
- Data Manipulations
- Scikit-Learn libraries
- Data Visualizations in Python
- Matplotlib, Seaborn, and GGplot
- Feature Engineering: Feature Selection and Extraction
- Model Selection
- Training, Testing and K-Fold cross validation
7. Introduction to Machine Learning
- Machine Learning
- Types of Machine Learning
- What is Supervised, Un-Supervised and Reinforcement
- What are the types of each learning technique
- Algorithms used in Machine Learning techniques
- Difference between Data Science, Machine Learning and AI
Generalized Linear Models (GLM)
- Introduction to generalized linear models
- Understanding of Linear and Logistic Regression
- Underfitting and Overfitting
- Trade-off between Bias and Variance
- Regularization techniques (Ridge, Lasso, Elastic-Net Regression)
- Ordinary Least squares
- Maximum Likelihood
- Sigmoid Function
- Cost Function
- Gradient Descent
- One-hot Encoding
- Label Encoding
- Model Evaluation metrics
- Feature Engineering (Features Selection, Extraction)
- R-Square, Adjusted R-Square, RSME
- Confusion Matrix
- Evaluation metrics (Precision, Recall, F-Score, Accuracy)
- Sensitivity and Specificity
- ROC-AUC curves
- Assumptions of Linear Regression
- Imbalanced Data
- Sampling issues- Over sampling and Under sampling
- SMOTE, ADASYN and Near Miss
Decision trees and Random Forests
- Introduction of Decision Tree and its applications
- Types of Decision Tree
- Terminologies in Decision Tree
- Pros and Cons of Decision Tree
- CHAID analysis
- Root nodes Identification
- Gini Index, Entropy, Chi-Square, Reduction in Variance
- Solution for overfitting in Decision Tree
- Tree pruning
- Hyperparameter tuning
- Random Search and Grid Search for auto selection of parameters
- What is Bagging?
- Introduction to Random Forest and its applications
- Importance of Random Forest
- Significant feature selection using Random Forest classifier
Boosting Machines
- Ensembling Techniques
- Bagging vs Boosting
- Gradient Boosting Algorithms
- Gradient Descent in Boosting Algorithms
- Gradient Boosting Machines, XGBoost, and AdaBoost
- Regression and Classification boosting techniques
- Stacking
- Pros and Cons of boosting Machines
8. Clustering
- Introduction to clustering
- K-Means clustering
- Elbow Method
- Hierarchical clustering
- Real time applications
Text Mining
- Introduction to Text Analytics and Text Mining
- Introduction to NLP
- Real time applications
- Extractingtext from files
- Data cleaning
- Introduction to NLTK library
- Count Vectorizer
- Understanding of Stopwords and regular expressions
- Stemming and Lemmatization
- Word Cloud
- N-grams
- Fuzzy String Matching
- Levenshtein Algorithm
- Jaro-Winkler Algorithm
- Cosine Similarity
- Named Entity Recognition (NER)
9. K-Nearest Neighbors (KNN)
- What is KNN and why do we use it?
- KNN-algorithm and regression
- Curse of dimensionality and brief introduction to dimension reduction
- Pros and cons of KNN
- KNN-outlier treatment and anomaly detection
Naïve Bayes and SVM
- What is Naïve Bayes
- Bayes theorem, Conditional Probability
- Real time applications
- Pros and cons of Naïve Bayes
- What is Support Vector Machines (SVM)
- Training time complexity
- SVM Classifier
- Hyperplane, margin and Kernel
- Hyperparameter tuning
- Linear and Non-Linear SVM
Dimensionality Reduction
- Introduction to Dimensionality Reduction and its importance
- Principal Component Analysis (PCA)
- Kernel PCA
- Singular Value Decomposition (SVD)
- Linear Discriminate Analysis (LDA)
- T-Distributed Stochastic Neighbor Embedding (t-SNE)
- Applications of Dimensionality Reduction
10. Time Series Forecasting
- Introduction to forecasting
- Data processing and indexing time
- Time Series & Time Series forecasting
- Understanding of Stats Models
- Auto Regressive Integrated Moving Average (ARIMA) model
- Components: Seasonality, Trend and Noise
- Autocorrelation
- Parameter Selection for ARIMA Time series
- Forecasting and Smoothing methods
- Forecasts Validation
- Simple moving average
- Exponentially weighted moving average
11. Deep Learning & Neural Networks
1. Neural Networks Architecture
2. Activation Functions a. Sigmoid b. Tanh c. ReLU d. Leaky ReLU
3. Forward & Backward Propagation
4. Understanding the Vanishing Gradient problem
5. Convolutional Neural Networks a. Filters/Kernels b. Convolution Operation for Edge detection c. Pooling & Zero padding
6. Understanding several CNN architectures a. AlexNet b. VGG16 c. ResNet d. Inception
7. Object Detection using YOLO
8. Siamoid v/s Softmax
9. Recurrent Neural Networks a. Tackling Long Range dependencies b. LSTM- Long Short Term Memory c. GRU
10. Seq2Seq Models for Machine Translation
11. Transformers a. Attention is all you Need b. BERT c. DistiIIBERT d. XLNet
12. Building Deep Learning Models using PyTorch
13. Tensorflow Framework
14. Tensors as MultiDimensional Arrays
12. Data Visualization Using Tableau
Introduction
- Installation of Trial Version of Tableau Public
- Design Flow
- Data Visualization
- Connecting Tableau to Various Data Sources
- Measures and Dimensions
- Colors, Labeling and formatting Exporting Worksheet
Advanced Concepts of Tableau
- Trend Line Analysis
- Dash Board Creation
- Formatting in tableau
- Forecasting using Exponential Smoothing
- Granularity and Trimming
- Seasonality
- Animations
- Assignment
Basics of Tableau
- A-B Ad-hic Testing
- Aliases
- Reference Line
- Anomaly Detection
- Sorts and Filters
- Time Series
- Chart Plotting
- Heat Maps
- Data Joining
- Data Blending
13. AWS For Data Science
- Why AWS For Machine Learning?
- Understanding several AWS services:
- a) KINESIS
- b) S3
- c) LAMBDA
- d) EC2
- e) REDSHIFT
- f) SAGEMAKER
- Setting up an S3 bucket to store data
- Practical Data Science with AWS Sagemaker
- Sagemaker Studio Notebooks
- Training and Evaluating an ML Model
- Deploying the Model
14. Big Data analysis with Spark
Introduction to Big Data analysis with Spark
• What is Big Data
• The 3 \Ps of Big Data
• PySpark : Spark With Python
• Understanding SparkContext
• Interactive use of Spark
• Loading data in Py Spark shell
• lambda() with map()
• lambda() with filter()
Programming in PySpark RDD’s
• Abstracting data with RDD’s
• RDDs from parallelized collections
• RDDs from external datasets
• Partitions in your data
• Basic RDD Transformations and actions
• Map and collect
• Filter and Count
• Pair RDDs in PySpark
• ReduceByKey and collect
• SortByKey and collect
• Advanced RDD Actions
• CountingByKeys
PySpark SQL & Dataframes
• Abstracting Data With Dataframes
• RDD to Dataframe
• Loading CSV to Dataframe
• Operating on DataFrames in PySpark
• Inspecting data in DataFrame
• Dataframe subsetting and cleaning
• Filtering your dataframe
• Interacting with dataframes using PySpark SQL
PySpark on Cloud
• Executing Spark programs on Azure
• Executing Spark programs on AWS
15. Chatbot Architecture
- NLP for Chatbot
- Understanding Rasa Framework
- Rasa NLU
- Named Entity Recognition (NER) using Spacy
- Intent Classification
- Rasa Core – Dialog Management
- Case Study : Application of Chatbots in Banking Industry
16. Capstone Projects
Choose from wide range of projects.
- Sentiment Analysis – Amazon
- Churn Prediction – Tele Communication
- Pneumonia Detection – Healthcare
- Fraud Detection – Insurance
- Predictive Maintenance – Microsoft
- Questioning & Answering – Chatbot
Digital Nest Advantage
Have a Great Journey of Learning at Digital Nest
Our Courses are taught by Real time Industry Experts with latest teaching tools and techniques ,Our LMS and dedicated Support manager are Key Important aspects that make learning easy and simple.
Interactive Learning
All the Courses taught by us are Interactive , with Limited strength in a batch we make sure that you get the best experience
Dedicated Placement Officer & Job Portal
We conduct Mock Interviews and Screen CV’s to make you industry ready. We have a unique job portal disclosing all the job listings posted by the recruiter
Network with Alumni
Digital Nest has over 6000+ alumnus working with various Organizations. We have a Networking app to connect with the alumni as well
Dedicated Program Manager
We have a dedicated Program Manager who will Constantly take feedback, for the Students to have a better learning experience
World Class Learning Management System
Digital Nest has built a Software for students to learn using LMS. Students can download material and freely access all the live and Recorded sessions with no hassle
Case Studies, Material , Assignments & Assessments
All our courses are not just Theoretical, We teach using Practical methods such as Simulation Exercises, Projects, Assessments etc
Tools You Will Master
Advanced Data Science tools used by Data Scientists in real time
Get 30%+ Salary Hike + Career Transition
Post Graduate Programme In Data Science will help in career transitions with better hike
Admission Process
Eligibility 1
Bachelor’s degree (10+2+3/4) or equivalent qualification in any discipline from a recognized University with a minimum 55% score.
OR
Eligibility 2
Students who have appeared for their final year degree examination can also apply, however, their admission will be provisional and will be confirmed only after producing the results.
Step 1: Application submission
Check your eligibility, pay the fees for the Digital Nest Admissions Test, and fill in the application form in detail and take the Admissions Test.
Step 2: Application review
All applications are thoroughly reviewed by the admissions committee. Shortlisted students will be called for a personal interview during which they can present their portfolios.
Step 3: Personal Interview
Each shortlisted applicant will be personally interviewed by the admissions committee. Qualified applicants will receive a letter confirming the admission to the Post Graduate Programme in Data Science
Application Fee : INR 1,000 /-
Admission Fee : INR 20,000/-
Tuition Fee : INR 1,00,000/-
Examination & Certification Fee: INR 30,000/-
Total Fee :
Post Graduate Program in Data Science: INR 1,50,000 + Taxes
No cost EMI’s available from Rs. 6,999/month
Education Loan : Education Loan In Partnership with Propelled
We have an excellent Accommodation for the students across the globe . Contact the Couse Consultant for more details regarding the stay at hyderabad.
Our
Alumni Testimonials
Rated Avg 4.5* Out Of 5 by 2000+ Learners
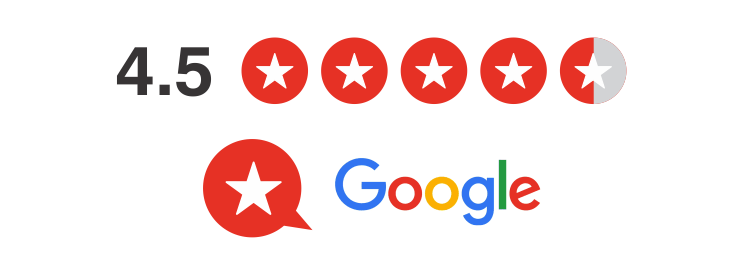
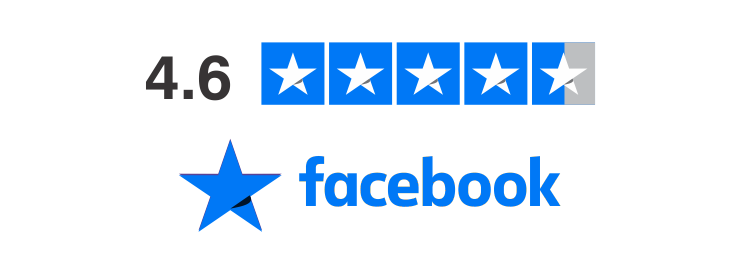
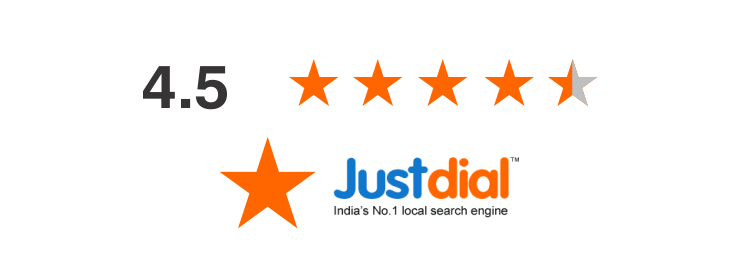
I am a 2017 pass out of B.tech. I took up many courses, but I could not get a job, then one of my friends suggested joining a data science course as there is a huge demand in the field. Then I came across Digital Nest in Hyderabad after a lot of research. This is the best Data Science institute in Hyderabad for learning Data Science Course. They have a power-packed curriculum that enabled them to learn through real-time practical examples and industry challenges. The trainers were extremely knowledgeable and gave insights into their real-time implementing challenges and experiences.
Though I come from non technical background, I never failed to ask doubts and participate in the assignments. The Digital Nest is a great platform, even for non technical students or slow learners like me. I feel so grateful for Digital Nest and specially their Data Science trainers for instilling so much confidence in me. I owe it all to Digital Nest for what I’m now. The practice, assignments and assessments tests are what really helped me grow. They have built a really good ambience, and house a really good team of counselors that help you through the whole journey. .
Digital Nest is one of the best Data science institutes in Hyderabad. I kept searching many institutes, and then I found Digital Nest, I approached and attended data science training demo, and then I realized that this is one of the best training institutes that I should choose. That’s where I began learning Data Science training at the Hitech city branch of Hyderabad. I must say that I’m very much satisfied with the trainers and curriculum.
I am a testing engineer. I came across an article in newspaper that data science and Data Science courses are in demand. Then I started searching in Google and I found Digital Nest. I was impressed by the demo session and I came to know various careers options. Then I thought of enrolling for this course and I am really satisfied and I would recommend as its one of the Best Data science training institute in Hyderabad
Our Students Hail From
Students , Working Professionals & Business Owners From Various Start Ups And MNC’s
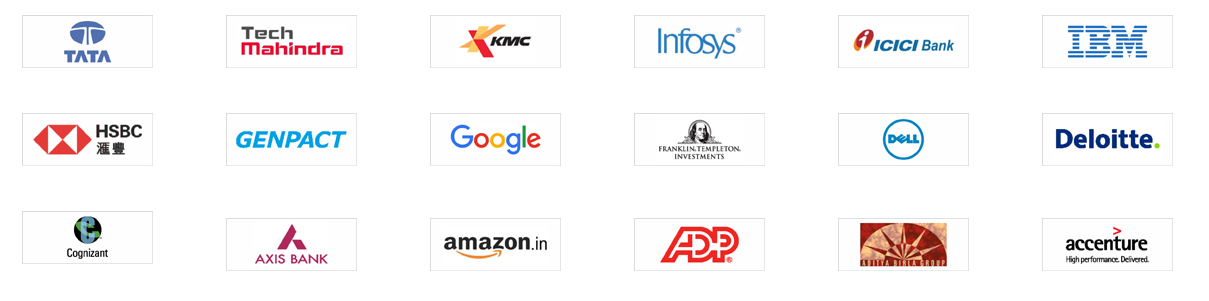
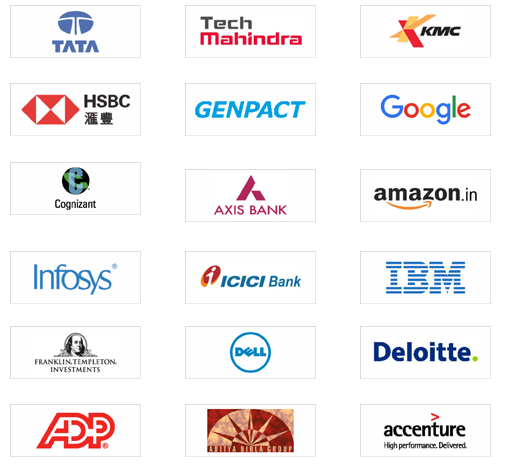
Curriculum
Post Graduate Program In Data Science syllabus is designed by alumnus of IIT Chennai and Subject Matter Experts
PG Certification Program in Data Science
Fee : INR 1,50,000/- + Taxes
Post Graduate Certification Program From
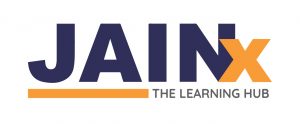
Options to choose either Classroom, Online Or Self paced Learning
320+ Learning Hours
Learning Using World Class Learnig Management System
6 + Projects
Dedicated Placement Manager for Interview Process
Access to the Instructor for doubts clarification during and after the course
100% Placement Assistance
Connect and Network with alumni, working with different organizations
Unique Job Portal to Access Jobs and Internships posted by Hr’s From Various Companies
FAQ’s
DS Program Details
-
What is the Post Graduation Program in Data Science?
Post Graduation Program in Data Science is a 11 month course curated for working professionals and freshers to gain deep knowledge and build a career in Data Science.
-
What is the eligibility criteria for this program?
This program is eligible for candidates who are in their final semester, recently passed out graduates and working professionals with 0-5 years experience.
-
Will I get any certificate after completion of the program?
You will be granted Post Graduate Program In Data Science Certificate from Jain University, Bangalore.
-
What is the duration of this program?
Post Graduation Program in Data Science is a 11 months course with 10+ hours of training per week. Classes will be held from Mon-Thur.
-
Will there be placements at the end of the program?
We will be providing placement assistance upon successful completion of the course. We have a job portal specially designed for placements. Candidates will be shown job openings in MNC’s and Startup’s for the roles such as Data Scientists, Data Analysts, Data Engineer, Machine Learning Engineer, etc.
-
Will I get any real-time projects from the program?
You will get your hands on 5 real-time projects under industry expert guidance.
-
Will I be evaluated during the program?
There will be continuous evaluation throughout the course. Evaluation will be done through assignments, quizzes, case studies, projects, etc
-
Who will be the faculty for this program?
Data Science modules will be taught by Industry leading experts with 8+ years of experience in Data Science.
DS Career Guidance & Support
-
Will I get any career guidance after the course?
Mentoring and guidance from Industry leading experts & trainers in Data Science will be given on how to build a resume. Career counselling will be given to find out the best career paths in Data Science. Interview preparation like mock interview sessions will be given during the end of the course.
-
How will my doubts be cleared?
Data Science trainer will be physically present during the class, where doubts can be cleared during the session. Doubt clearing sessions will also be held every week.
-
Will I get any back up classes if I miss any session?
We have a unique Learning Management System where every candidate can check the back-up classes if they miss any session. You can also check the assignments, materials in the app.
DS Admission process & Fee related queries
-
What is the Admission Process for this program?
Step 1: Application submission
Check your eligibility, pay the fees for the Digital Nest Admissions Test, and fill in the application form in detail and take the Admissions Test. The test is designed to assess creativity, aptitude, communication, acumen, general knowledge to gauge the applicant’s vision & interest in Data Science.Step 2: Application review
All applications are thoroughly reviewed by the admissions committee. Shortlisted students will be called for a personal interview during which they can present their portfolios.Step 3: Personal Interview
Each shortlisted applicant will be personally interviewed by the admissions committee. Qualified applicants will receive a letter confirming the admission to the Post Graduate Program in Data Science -
Should I have any minimum percentage to get enrolled in this program?
Applicants should have minimum 55% aggregate from a recognized University.
-
What is the fee structure for this program?
Application Fee : INR 1,000 /-
Admission Fee : INR 20,000/-
Tuition Fee : INR 1,00,000/-
Examination & Certification Fee: INR 30,000/-
Total Fee :
Post Graduate Program in Data Science: INR 1,50,000 + Taxes
No cost EMI’s available from Rs.6,999/month -
Can I pay through Credit Card EMI?
Yes, our payment gateway partner is Razopray, All the leading banks can avail the credit card EMI option with no hassle
-
Is there any refund policy for this program?